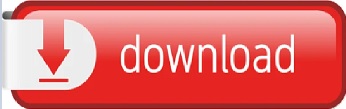

In our experiments, the centre-most layers of autoencoder models were re-trained with limited new data belonging to a different device. We hypothesized that devices of similar nature would have similar high level feature character- istics represented by the initial layers of the autoencoder, while the more distinct features are captured by the innermost layer of the neural network. This work explores the “transfer- ability” of a pre-trained autoencoder model across devices of similar and different nature. With IoT devices rapidly proliferating, training anomaly models on a per device basis is impractical. Machine Learning based anomaly detection ap- proaches have long training and validation cycles.
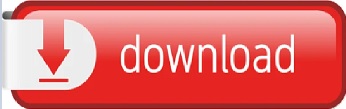